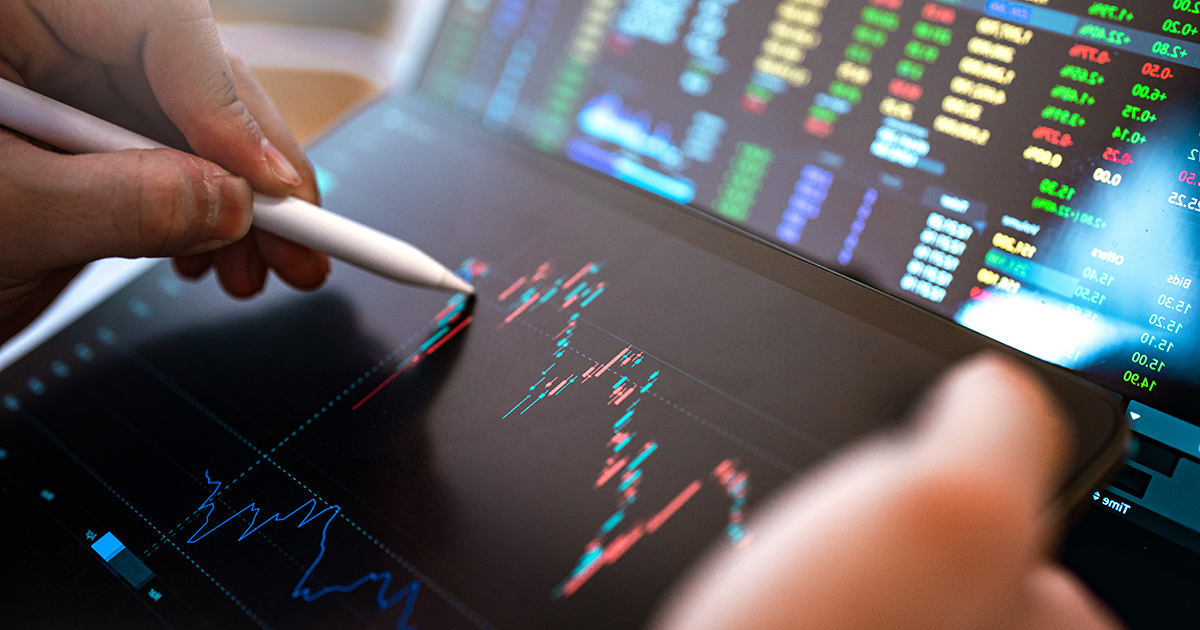
We think of technology less as a sector and more as a secular force that will affect every corner of the economy, generating opportunities for wealth creation (and destruction).
Every decade, a technology platform shift is accompanied by a major disruption in tech leadership. As these shifts play out, some businesses can sustain their leadership, but they are few and far between. The strongest companies today can be disrupted if they fail to capture the next platform or lose market share in their core business (Exhibit 1).
In November 2020, the U.S. Equity Growth team published a paper in which we hypothesized that artificial intelligence (AI) would not only define the next era of computing but would remake every sector of the economy.
Studying breakthroughs in machine learning and parallel computing architectures strengthened our conviction in that view. We have also been speaking directly with practitioners who are creating the future. A defining moment came in 2016, when we interviewed a pioneer in the field who said, “Computers can now see and hear with human-level accuracy – think about that.”
We did think about that, and imagined scenarios that are top of mind today. These include AI-driven step-change improvements in personalized assistants, health care diagnosis, autonomous driving and vision-enabled robotics, and an enabling layer of next-generation hardware and software infrastructure. Technology leaders are moving from imagination to investment, and they continue to play a key role in our portfolios.
As we consider the various ways that AI might transform the economy over the coming decade, we believe we are at a critical inflection point: AI will be a catalyst for significant investment and associated growth across hyperscalers (leading cloud service providers), infrastructure, hardware, software and many adjacent industries.
In this article, we discuss three areas of focus: the power of ChatGPT and large language models (LLMs), how technology infrastructure will change, and why AI’s impact will be broad. We also examine how investors might weigh their potential.
ChatGPT: “The iPhone moment”
Innovation tends to progress in a series of S-curves where advancement can happen very quickly. Today, generative AI is experiencing its own sharp rise. ChatGPT and large language models (deep neural networks trained on vast data sets) are providing answers to questions so accurately that their responses are often indistinguishable from experts. Nvidia co-founder Jensen Huang captured it best as an “iPhone moment” – a point where technology becomes so immediately useful that its adoption curve accelerates for both consumers and businesses.
As many investors understand, LLMs work by probabilistically predicting the next word in a sentence. Some might be tempted to call this a statistical trick, but what is radical about this technology is that in pursuing accurate next-word predictions, LLMs exhibit an increasingly deep understanding of reality.
As OpenAI’s chief scientist, Ilya Sutskever, explains: “To learn statistical correlations in text, what the neural network learns is some representation of the process that produced the text. This text is actually a projection of the world.” More simply, by training on a corpus of data so vast that no human could ever read it all in multiple lifetimes, the most advanced LLMs have built foundations to reason about the world.
While models like GPT-4 have not been trained to be experts in any particular field, they find themselves strong in multiple fields: translation, law, music, coding, chemistry, finance – the list is long. They can do considerably more than text output, including, for example, understanding and creating images. Prompted by a brief description of a scene or action, generative AI models such as Midjourney and Adobe’s Firefly can create images as realistic as any photo.
In the future, models that train on a combination of media, from text to video, will likely develop more powerful reasoning capabilities. When augmented with proprietary data or fine-tuned for use in a specific sector, LLMs may reason at an elevated level. Looking ahead, we see potential for AI agents to autonomously self-improve and even participate directly in the economy, achieving business goals.
The next three years of infrastructure
As AI accelerates the diffusion of technology across the broader economy, we envision a new computing infrastructure that can enable consumer and enterprise adoption. This will not only support demand for existing hyperscaler AI applications but also encourage new application development by a community of AI-focused entrepreneurs.
Already, capex spending for new AI computing data centers is rising at a rapid pace (Exhibit 2). Accelerated spending by hyperscalers should grow the opportunity set by about 10x and equate to annual capex spending that could be as high as USD 1 trillion within the next 10 years.
Often, when a major platform shift occurs – think mainframes to minicomputers, PCs to mobile devices, CPUs to GPUs (central processing units to graphics processing units) – it creates entirely new pools of demand. In the next few years, we expect a record pace of new, densely packed, high powered data center builds. These will be filled with GPUs, custom silicon, advanced memory packages and photonics to support network bandwidth.
At the same time, an aggressive investment in software infrastructure will be required to address specialized needs such as training with private data, real-time updates and security tools. Software and LLMs will likely decouple over time as infrastructure software providers leverage their expertise to better control data accessibility and protect privacy. Companies will customize AI to fit their specific corporate culture and business needs. In other words, a theme park operator will build software infrastructure that is very different from that of a large insurance provider.
Who will lead the coming era of innovation?
Given the importance of proprietary data, existing customer reach and the high costs of training and deploying LLMs, some argue that the most well-resourced companies are best equipped to profit from generative AI. That is one potential outcome, to be sure, and an easy argument to make as mega caps such as Microsoft, Google and Nvidia take turns leading the charge in AI development.
But that framing misses a key element: AI usage trends are set to explode as more efficient forms of data consumption are introduced. Even within the supposedly zero-sum example of Microsoft vs. Google in the search sector, early signs suggest that overall queries are accelerating for every company. As technology boosts asset efficiency, profit pools get wider and deeper.
What’s more, improvements in semiconductor efficiency and optimized software naturally democratize building and running LLM models, driving AI to the mass enterprise market and growing the roster of its potential use cases. As this happens, deployment methods can also change, with smaller or more local clouds emerging that are dedicated entirely to AI workloads.
In addition, efficiencies in technology infrastructure can lead to more open-source development. Open source can in turn act as a default option for developers looking to push back against AI centralization, and enterprises looking to partner with more transparent AI models.
A time for “strong opinions, loosely held”
In an environment of rapid change and meaningful unknowns, humility and iterative thinking are essential. Within the AI community, practitioners actively debate the trajectory and timing of AI advancements while raising questions about core infrastructure choices, the limits of LLM model size and the search for efficacious data that models can train on.
Given these unknowns, we have to appreciate that as technology advances and clarifies existing imaginations, new imaginations, now too obscured to fully understand, will take flight. While we can see the power of AI with more clarity since its “iPhone moment,” we need to recognize that this is a time for “strong opinions, loosely held” (a nod to futurist Paul Saffo’s “strong opinions, weakly held”). We must be agile even if we believe generative AI can spur an aggressive investment cycle.
Humility is imperative, especially in the software sector, where there can be extreme outcomes. There may be one application to rule them all (in customer relationship management or enterprise resource planning, for example). Or perhaps there will be an extreme splintering of applications in which each enterprise develops its own custom software. Both dynamics are in play in the development of language models as hyperscalers work on their own proprietary systems while others (including Meta) advance open-source deployments.
When Apple had its iPhone moment in 2007, it set off a series of incremental yet meaningful iterations in the mobile phone market. Those iterations made mobile phones more useful and deepened our historical understanding of what made Apple’s innovation so powerful. As waves of innovation moved in a cycle of decentralization and centralization (mobile computing is decentralized, the cloud is centralized), entirely new industries were created. We think the same process is now underway in the wake of ChatGPT. When we look back in 10 years, we expect to see profound change.